Abstract
Mathematical risk assessment models based on empirical data and supported by the principles of physics and engineering have been used in the insurance industry for more than three decades to support informed decisions for a wide variety of purposes, including insurance and reinsurance pricing. To supplement scarce data from historical events, these models provide loss estimates caused to portfolios of structures by simulated but realistic scenarios of future events with estimated annual rates of occurrence. The reliability of these estimates has evolved steadily from those based on the rather simplistic and, in many aspects, semi-deterministic approaches adopted in the very early days to those of the more recent models underpinned by a larger wealth of data and fully probabilistic methodologies. Despite the unquestionable progress, several modeling decisions and techniques still routinely adopted in commercial models warrant more careful scrutiny because of their potential to cause biased results. In this chapter we will address two such cases that pertain to the risk assessment for earthquakes. With the help of some illustrative but simple applications we will first motivate our concerns with the current state of practice in modeling earthquake occurrence and building vulnerability for portfolio risk assessment. We will then provide recommendations for moving towards a more comprehensive, and arguably superior, approach to earthquake risk modeling that capitalizes on the progress recently made in risk assessment of single buildings. In addition to these two upgrades, which in our opinion are ready for implementation in commercial models, we will also describe an enhancement in ground motion prediction that will certainly be considered in the models of tomorrow but is not yet ready for primetime. These changes are implemented in example applications that highlight their importance for portfolio risk assessment. Special consideration will be given to the potential bias in the Average Annual Loss estimates, which constitutes the foundation of insurance and reinsurance policies’ pricing, that may result from the application of the traditional approaches.
You have full access to this open access chapter, Download chapter PDF
Similar content being viewed by others
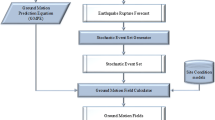
1 Introduction
Since the early 1990’s the use of catastrophe risk models has been adopted in the insurance industry to estimate the likelihood of observing losses in a given period of time due to the occurrence of natural events, such as earthquakes, tropical and extra-tropical cyclones, and floods. Too few and scarcely representative historical loss data were available to support a robust estimation via traditional statistical techniques or expert judgment, especially for large events that were not yet observed in recent historical times. In essence these probabilistic catastrophe risk models, which are based on applying the best available science on the existing data, were used to simulate virtual loss observations to augment scarce or missing real loss observations. Since their advent, these models have been used by insurance/reinsurance companies, rating agencies, hedge funds, catastrophe risk pools, mortgage lending institutions, governments and corporations, among others, for all sort of risk management decisions. One such a decision involves setting the premium of insurance/reinsurance policies for single assets and portfolios of assets. We will dwell on insurance pricing towards the end of this chapter.
All these catastrophe risk models have all the same structure and include four modules regardless of which natural event they are meant to address, only the details differ from one peril to another.
Firstly, an exposure module, which describes all the characteristics of the assets at risk in the region of interest. These assets usually include all the building inventory and, sometimes, infrastructures. In many insurance applications, however, the exposure is not the portfolio of the entire built environment but simply the specific portfolio of assets to be insured or reinsured. Each asset in the portfolio is traditionally assigned to one of the many different classes of structures (e.g., midrise reinforced concrete frame buildings of the 1970’s era) that exhibit a different level of vulnerability to the natural events under consideration.
The second is the hazard module, which is conceptually divided into two parts: an event occurrence sub-module, which defines the rate of occurrence of future events in terms of size and location. This sub-module is responsible for producing stochastic catalogs of simulated future events that are statistically consistent (but not identical) to those occurred in the past. The second sub-module deals with the predictions of the effects that each event in the stochastic catalog may cause in the nearby region. The effects may be, for example, ground motion for earthquakes, or wind, storm surge, and precipitation for tropical cyclones. For example, in the case of earthquakes, given the occurrence of an event of given magnitude, M, this sub-module provides the distribution of the intensity measure (IM) for any site at a given distance, R, from the rupture with local soil characteristics, often conventionally expressed in terms of the average shear wave velocity, Vs30, in the top 30 m.
The third is the vulnerability module, which contains for all different types of assets the relationships that provide the level of loss ratio (namely a loss expressed as a percentage of the total replacement cost of the asset) and its variability expected for any given intensity level of the effect that the asset may experience (e.g., for Peak Ground Acceleration of 0.05 to 3.0 g in the case of earthquakes or wind speed from 50 to 300 km/h for tropical cyclones) in its lifetime. These relationships that associate an IM of the effect of the event with a loss ratio are called vulnerability functions. Vulnerability functions are often constructed by engineers by convolving two other types of functions: fragility functions and consequence functions. Fragility functions, which are derived for specific damage states (ranging say, from minor damage to collapse), provide the likelihood that an asset will end up in any given damage state should it experience any given level of IM. Consequence functions simply link the damage states to distributions of loss ratios (e.g., a minor damage state may correspond to losses in the 2 to 5% of the replacement cost of the asset). Both vulnerability and fragility functions will be discussed later in the chapter.
The fourth and last is the Loss Module, which handles the computations of losses for each event in the hazard module and for each asset in the exposure module by applying the asset-class-specific vulnerability function as specified in the vulnerability module. The losses could be the so-called ground-up losses, which include all the losses that one would need to sustain, for example, to repair or replace all the structural and non-structural components damaged by an event, or the insurance losses. The latter are computed from the former by applying the policy conditions (e.g., deductibles and limits). Note that the monetary losses may in some cases not refer to repair cost but to costs incurred due to downtime, i.e., the time required to make the asset functional again. In other applications, the losses computed are non-monetary and are measured in terms of number of injuries or fatalities caused by the damage and collapse of the assets caused by the event. For monetary losses, the standard outputs of the Loss Module are the Average Annual Loss (AAL) and the so-called Exceedance Probability (EP) Loss Curve. The AAL is the expected loss that the stakeholder can expect to pay, on average, every year over a long period of time. If the stakeholder is, say, the owner of a building the AAL is the amount of money that, on average, is needed every year for fixing the damage caused by natural events. If it is an insurance company, the AAL is the amount of money that, on average, the insurance is expected to pay the insured every year because of the damage caused by natural events. The EP Loss curve provides the annual probability (or rate) that the losses (by single event or aggregated for all the events in a year) will exceed different amounts of monetary values. These losses may refer to a single asset or, in the case of an insurance or reinsurance company, to a portfolio of many assets. These two standard outputs are complementary and fully consistent with each other.
In the rest of the chapter we will discuss some of the caveats of the traditional methodologies applied in commercial catastrophe risk models for assessing earthquake risk. The next section will deal with the event occurrence part of the hazard module and will be followed by a section that will address the shortcoming of the universally adopted approach for deriving vulnerability functions for classes of buildings. We show two applications where we propose enhancements to the current methodologies that we believe are ready to be embraced in the next generation of catastrophe risk models. Then we will discuss the impact on seismic hazard and risk due to the next generation of non-ergodic ground motion prediction equations. This enhancement, which pertains to the second part of the hazard module, is indeed very promising and will certainly revolutionize the ground motion prediction of earthquake models of the near future. However, this new approach is more complex and requires an amount of data that is nowadays only available in very few parts of the world. Therefore, its widespread adoption is still premature and will need additional data and research before it is incorporated in commercial earthquake risk assessment models. Finally, we will show how the potential biases in the loss estimates caused by the caveats of the traditional approaches adopted in the current commercial models may affect insurance pricing for earthquake risk.
2 Should Earthquake Sequences be Removed from Seismic Hazard and Risk Assessment Models?
Probabilistic Seismic Hazard Analysis (PSHA) is the methodology universally adopted in the hazard module of all earthquake risk assessment models in use today. In the large majority of PSHA studies and in all those used for loss estimation purposes, the rates of occurrence of events have been derived from historical and instrumental seismicity catalogues that undergo a so-called “declustering” procedure. This procedure involves identifying earthquake clusters (in time and space) and removing from the catalogues all but one event per cluster (typically the one with the largest magnitude). The earthquakes kept are classified as “mainshocks”, while those that are discarded are referred to as “foreshocks” or “aftershocks”, depending on whether they occurred before or after the mainshock, respectively. In some clusters this procedure removes also “triggered” events that did not break the same part of the fault ruptured by the mainshock as, loosely speaking, “proper” foreshocks and aftershocks do, but adjacent fault segments or, sometimes, different nearby faults altogether. Hereafter, for brevity we will refer to all these removed events simply as aftershocks. The rationale behind this practice is to ensure that the occurrences of earthquakes in the final catalogue are independent events. This convenient property in turn allows the analyst to employ the well-known Poisson process to model the occurrence of future (mainshock-only) seismicity with rates derived from the declustered historical catalogues. The clear advantage of this choice is the mathematical simplicity of the modeling process. On the other hand, one can expect the resulting seismic hazard to be underestimated due to the exclusion of non-mainshock events whose ground motions could exceed significant levels of intensity at the site(s) of interest.
The underestimation of hazard caused by the practice of considering only mainshocks may be less severe in the small minority of advanced PSHA studies that rely on fault (rather than area source) source characterization for which earthquake rates are computed strictly from geologic and geodetic data. In these very few cases, the earthquake activity rate on a fault is computed by balancing the long-term seismic moment build up estimated by geologic or geodetic observations and the seismic moment available for release by future earthquakes. Hence, it may be less critical whether the moment-rate is balanced by only mainshocks of larger magnitude or by entire sequences of events. Enforcing the moment balance using sequences is, however, clearly the preferred approach.
In the early days of PSHA, reducing the phenomenon of seismicity to just mainshock events might have been warranted to facilitate its implementation in the state of practice and also somewhat inevitable given the scarcity of the then-available seismicity data and the limited understanding of the problem. Moreover, the primary application of PSHA at the time was to underpin seismic design codes by defining the ground motion input that a given structure was expected to withstand with a given likelihood within its lifetime. In that context, declustering was largely justified by the appealing, but imprecise, notion that if a structure is designed to withstand the ground motion of the “stronger” (in magnitude) mainshock, it will also be able to survive that of the “weaker” aftershocks. Or, to the very least, if the structure survived the mainshock then the occupants could leave unharmed before the aftershocks would increase the severity of the damage and perhaps destroy it altogether.
This somewhat naïve approach, which was to a certain extent justifiable for that application, has, however, been carried over to earthquake risk modeling. This approach is still routinely followed until the present day, as if all the events in a sequence but the mainshock would cause no additional damage, no further loss of serviceability of buildings, and no additional repair cost. In the insurance industry, for example, to legitimize this simplistic approach it is often reasoned that the effects of aftershocks and triggered events are already implicitly accounted for since the employed vulnerability curves are calibrated to match actual damage data gathered after the entire earthquake sequence has taken place. However, aside from the scarcity of such damage and loss data that only seldom allow the development of empirical vulnerability functions, the validity of the claim that the effects of aftershocks and triggered events can be embedded in conventional vulnerability curves is not only questionable but also lacks a theoretical foundation. Furthermore, even if the claim were true, this approach would imply that using these somewhat “inflated” vulnerability curves would produce overestimated losses in all those cases where no major aftershocks are triggered after the mainshock earthquake.
In an attempt to bring to light potential sources of bias, several shortcomings of the current mainshock-only view of seismicity are outlined in the following paragraphs.
2.1 Fewer Earthquakes Modeled
The most obvious deficiency—yet hidden in plain sight—is rooted in the main argument for declustering: the expectation that the mainshock earthquake will always be more damaging than its preceding and succeeding lower magnitude events. While generally true, it does not necessarily apply for every structure in the region affected by the seismic cluster. To demonstrate this point, let us look at the potential ground motion intensity that different earthquakes in the same cluster can induce. As an example, Fig. 11.1a and b show the peak ground acceleration (PGA) ShakeMapsFootnote 1 provided by the Italian Institute of Geophysics and Volcanology (INGV) for the two M5.9 and M5.8 events in the Emilia-Romagna sequence that struck this region of Italy in May 2012. Figure 11.1c, on the other hand, shows a map of the maximum PGA value at each grid cell from the two earthquakes. It is clear from these plots that there are areas in which the largest expected PGA comes from the first event and areas where the largest expected PGA is induced by the second event. This is arguably a consequence of the distance of these sites to each of the two ruptures, but it could also be partly attributed to potential differences in the source or path effects that determine the waveforms. Nevertheless, catastrophe risk models consider only one of these events (in this case the first while the second is removed) for the computation of seismicity rates. This means that to replicate the damage potential of this sequence the stochastic catalog of these models will include only one event whose ground motion field covers a smaller area than that actually affected by the sequence. In other words, in the Emilia-Romagna example the stochastic catalog may include a simulated event whose footprint of the ground motion used for damage and loss computation will be similar either to Fig. 11.1a or b but never to Fig. 11.1c. Hence, this approach will inevitably fail to take into account the contribution of foreshocks/aftershocks in the estimated maximum ground motion experienced at each site.
Another example that stands out is the 2016–2017 Central Italy sequence, which comprised thousands of earthquakes (11.2a), including nine M5 + earthquakes. Yet, if one were to apply a standard declustering procedure, such as the one proposed by Gardner and Knopoff (1974), the only significant event retained would be the October 30 M6.5 Norcia earthquake. In other words, as far as traditional seismic risk assessment is concerned, the August 24 M6.0 Amatrice earthquake, the October 26 M5.9 Visso earthquake and the six additional events with moment magnitude above 5 never happened, even though the damage they caused is well documented in Sextos et al. (2018) and also shown here in Fig. 11.2b. Another striking example outside of Italy is the 2010–2011 Canterbury earthquake sequence, which was started by the M7.1 Darfield earthquake followed by three large aftershocks with magnitude greater than 6 that devastated Christchurch more than the mainshock did.
a Epicenters of events belonging to the 2016–2017 Central Italy sequence, and b percentage of buildings in the town of Amatrice in different damage states immediately after the 24th August earthquake and after the entire sequence (Stewart et al. 2017a)
In summary, from the viewpoint of single-site seismic hazard (and risk) assessment, what was stated above means that even though one can arguably claim that the rate of clusters is correctly estimated by counting mainshocks, the probability of ground motion exceedance given a mainshock rupture is most likely underestimated; its assessment does not factor in the impact of non-mainshock earthquakes unless, to a certain extent, a fault-specific geologic/geodetic approach based on seismic moment balance is followed. This results in seismic hazard (and risk) estimates that are biased low. This conclusion obviously carries to portfolio risk assessment. Therein, the spatial distribution of the exposed assets interestingly implies also that if a sequence involves multiple strong earthquakes, it is very likely that different assets at different sites are primarily affected by different earthquakes within the same cluster. Including all events in a sequence increases the number of potentially affected structures and enlarges the spatial footprint of damage.
2.2 Damage Accumulation
A different, more intuitive and perhaps more widely understood limitation of the current mainshock-only state-of-practice pertains to the so-called damage accumulation phenomenon. It is generally expected that structures in pre-existing damaged conditions owing to previous earthquakes are more prone to further deterioration, even if the following ground motions are weaker than the previously experienced ones. As a result, sites that experience more than one significant ground motion, i.e. sites within or in the vicinity of the epicentral areas of multiple events of a given sequence, are likely to experience losses that are higher than the losses that would be inflicted by the ground shaking of any of these events individually. The aforementioned Fig. 11.2b shows the percentage of buildings in the town of Amatrice that were assessed to be in damage states ranging from 0 (no damage) to 5 (collapse) during inspections just after the first significant 24th August event, as well as at the end of the entire 2016–2017 sequence, as reported by GEER (Stewart et al. 2017a). Even though the strongest ground motion in Amatrice was recorded during the initial 24th August shock, it is evident that the cumulative loading experienced during the sequence aggravated the condition of the building stock and even pushed a significant amount of already damaged buildings to collapse.
2.3 Arbitrariness in Declustering and Its Unintended Consequences
Aside from such conceptual defects of the mainshock-only view of seismicity for risk assessment purposes, there are additional motives to seek solutions to move past it. For instance, the process of declustering is highly subjective. The choice of the declustering algorithm that is incumbent on the analyst can lead to more or fewer earthquakes flagged as mainshocks, which in turn can result in strikingly different seismicity rates (Stiphout et al. 2011). Even within the boundaries of the same declustering technique, different analysts may choose different values of the parameters to delimit the spatio-temporal window for sifting earthquakes, which would lead to different numbers of retained earthquakes and, therefore, to different estimates of mainshock seismicity rates. Moreover, a declustering scheme that keeps the largest magnitude event of each cluster may lead to an unintended distortion of the magnitude-frequency distribution (Marzocchi and Taroni 2014). The latter is generally thought as exponential (Gutenberg-Richter law) for non-declustered catalogues, yet this shape is not fully preserved after declustering. The reason for this potential distortion is that largest events are likely maintained (as mainshocks) while lots of smaller magnitude events are filtered out. If an exponential function is then fitted to this modified catalogue, an underestimation of the b-value may take place. Smaller b-values in general lead to overestimation of hazard.
An additional concern regarding declustering is whether it really achieves its main purpose, i.e. to render the catalogue Poissonian (Luen and Stark 2011; Stiphout et al. 2011). Lastly, modeling seismicity as a time-independent mainshock-only process bears important practical limitations too. A particularly critical one is the inability to produce credible seismic hazard and risk estimates in periods of elevated seismic activity, such as during ongoing sequences or swarms of seismicity. Conventional time-independent, mainshock-only models are unable to pick up the increased likelihood of a new large earthquake and would yield unaltered loss predictions. This time independence of earthquake occurrence is acceptable, and also somewhat desirable, when the resulting seismic hazard is applied for underpinning design provisions in building codes that remain in force often for a decade or more and cannot change any time an earthquake occurs during the time they are adopted. But it is limiting in applications aiming at insurance and reinsurance pricing, where one is strictly interested in assessing risk for a short to mid-term time frame. Given that productive seismic sequences following large mainshocks may last for many years, this can render the results of seismic risk models deficient, if not unusable, for a significant amount of time.
2.4 Including Earthquake Sequences in Seismic Risk Assessment
Motivated by the above, there has been an increasing effort in recent years to commence quantifying the potential impact that foreshock, aftershock and triggered events have on seismic risk estimates. Some authors have attempted to assess the long-term seismic risk of individual buildings accounting for aftershock sequences (Jalayer and Ebrahimian 2016; Shokrabadi and Burton 2017), while a few have even tried to investigate the impact of seismicity clustering on short- and long-term portfolio risk assessment (Field et al. 2017; Papadopoulos and Bazzurro 2021; Shokrabadi and Burton 2019; Zhang et al. 2018). Figure 11.3 shows exceedance probability loss curves from the study of Papadopoulos and Bazzurro (2021), derived for the building stock of Umbria in Central Italy using a standard Poisson model, as well as representing seismicity via the Epidemic-Type Aftershock Sequence (ETAS) model (Ogata 1998). Given that the latter is a time-dependent model, its earthquake rates vary significantly depending on the past seismicity that is used as initial condition. The curves presented in the left panel of Fig. 11.3 refer to a one-year “active” period starting from 26/04/2017, i.e. about 8 months after the onset of the 2016–2017 Central Italy sequence, but only about three months after the last four M > 5 events. Thus, it constitutes a period of increased seismic activity, which translates to significantly amplified risk estimates. On the other hand, the curves on the right panel refer to a random year of seismicity; therein the amplification of losses (due to the consideration of sequences) is still important, but clearly not as pronounced as in the former case.
Comparison of annual exceedance probability loss curves for the residential building stock in Umbria, obtained using a Poisson recurrence model and an ETAS model (adapted from Papadopoulos and Bazzurro (2021)). The left panel shows results referring to a specific active period following the 2016–2017 Central Italy sequence as described in the text, while the right panel refers to a random year of seismicity
Moreover, a first attempt to capture the effects of damage accumulation was also made. Damage-dependent fragility models, i.e. fragility functions referring to buildings with initial damage, were developed for a set of building classes by means of nonlinear single-degree-of-freedom (SDOF) system representations. For every rupture in the 20,000 realizations of the 1 yr-long stochastic catalogues used in the analyses, after the first event the damage states of the assets in the exposure dataset were sampled, stored and used as initial conditions for the damage estimation for the next event in the catalogue, if any. Given that each catalog covers only 1 year of seismicity, the assumption that no repair actually occurs in between earthquakes is certainly tenable. An alternative simplified computational workflow was also explored in which the same fragility curves were used regardless of the damage and loss sampled in previous events (but care was taken to avoid double counting losses by keeping track of the loss ratio of each building asset from previous earthquakes during the same year, if any). The loss curves obtained by explicitly accounting for damage accumulation and using the aforementioned simplified approach are shown in Fig. 11.3 in blue and cyan respectively, and compared against loss curves derived following a mainshock-only Poisson process based model. For more details on the loss estimation framework, the reader is referred to Papadopoulos and Bazzurro (2021). As expected, when accounting for damage accumulation the computed losses were found to be higher (Fig. 11.3), but its impact is smaller than that due to the consideration of earthquake clusters in risk assessment. This limited difference is due to the spatially limited effects of damage accumulation, which are significant only in the epicentral area of the largest event where many already severely damaged buildings are left to cope with additional shaking from other events in the sequence. In areas farther from the epicenter, the average damage level is low and the vulnerability to ground motion caused by successive earthquakes is essentially unchanged. Note that these findings cannot be generalized without further research and that the simplistic modeling of the complex phenomenon of damage accumulation may be somewhat coloring the impact of the phenomenon. However, the trend is clear.
In any case, while there is still no wide consensus on the methods and practices to utilize for modeling the spatio-temporal clustering of seismicity, most of the early studies suggest that aftershock sequences can have a moderate to large effect on seismic risk estimates. These early findings should arguably prompt researchers and catastrophe risk modelers to interpret and use the results of traditional risk models with caution. On the other hand, they also highlight the need for continued research to improve our capacity to more accurately capture the effects of seismicity clustering and begin refining earthquake risk models accordingly.
3 Why Identical Buildings at Different Locations have Different Vulnerability?
Vulnerability functions play a central role in regional seismic loss assessment for portfolios of structures (Calvi et al. 2006; Rossetto and Elnashai 2003) and are the main result of the vulnerability module of earthquake risk models. Based on the desired accuracy as well as the availability of the required data, four different approaches have been utilized for the development of such functions for portfolio loss estimation: (1) expert judgment, (2) empirical, (3) analytical/mechanical, and, (4) hybrid (e.g., empirical plus analytical) (Kappos et al. 1995; Kappos et al. 1998; Barbat et al. 1996; Akkar et al. 2005; Bommer and Crowley 2006) methods. The first approach, widely used in the early days (ATC-13 1985; Brzev et al. 2013), has been abandoned, in the sense that it is no longer used in isolation but only to validate the reasonability of the results derived using other approaches. The second approach uses damage data collected in damage reconnaissance missions after an earthquake and loss data from insurance claims to generate vulnerability functions (Orsini 1999; Rossetto and Elnashai 2003; Di Pasquale et al. 2005; Porter et al. 2007; Straub and Der Kiureghian 2008; Rossetto et al. 2014; Noh et al. 2015). This is usually the preferred route provided that enough usable damage/loss data are available for a specific class of structures (e.g., midrise reinforced concrete frames of the 1970s) and that the ground motion experienced at the sites where the damaged structures are located can be estimated with a reasonable accuracy. This approach, however, is seldom practicable and even when it is, it is only applied to some specific building classes (e.g., wood frame buildings in California) because post-event specific data for each building class are never collected in such a clean fashion to make it applicable across the board. To the authors’ knowledge the second approach has never been applied alone to all construction classes in any earthquake risk assessment model without resorting to some support from analytical studies. In the third approach, numerical analyses (Kennedy and Ravindra 1984; Porter et al. 2014; Silva et al. 2015; D’Ayala et al. 2014) carried over on computerized models of representative archetype (FEMA-P695 2009) or index buildings in each class provide simulated losses to replace, or supplement, missing or scarce empirical data. Of course, there are numerous cases, for example with newer buildings that never experienced a damaging earthquake, where clearly the analytical approach is the best, if not the only, viable option.
3.1 Vulnerability Functions based on the Analytical Method
In applying the analytical method, which is by far the most widely adopted in practice, for each class of buildings (or other types of structures, such as bridges) engineers generate finite element models of one or more structures using either a nonlinear single-degree-of-freedom (SDOF) or a multi-degree-of-freedom (MDOF) representation. Consequently, based on the required level of accuracy, structural analysis and vulnerability analysis methods are employed to assess their response to different levels of ground motion. The utilized structural analysis approaches are either nonlinear static analysis such as capacity-spectrum (FEMA 2003; Sousa et al. 2004; Calvi and Pinho 2004) plus displacement-based methods (Pinho et al. 2002; Restrepo-Vélez and Magenes 2004) or nonlinear dynamic analysis (Haselton et al. 2011; Jayaram et al. 2012; Silva et al. 2014), which typically uses ground motion recordings from past earthquakes, as discussed later. In modern, last-generation models, however, the simpler but more approximate nonlinear static analysis has been largely abandoned in favor of its more accurate dynamic counterpart. Hence, in the following we only focus on the issues related to using the nonlinear dynamic analysis for developing analytical vulnerability functions.
Vulnerability analysis approaches use a single vulnerability function for assessing the performance of the entire building. The building performance is measured either by means of global response metrics, or by looking at the performance of each specific building component. In the latter case, the response of a component depends on its location and, therefore, it is gauged by story-specific response metrics, (Porter et al. 2001; Mitrani-Reiser 2007). The component-based methodology (FEMA-P58 2012), whose applications can be found in several studies (Porter et al. 2001; Mitrani-Reiser 2007; Kohrangi et al. 2016b), is arguably superior but it is more time consuming because it requires detailed information about the location, the damageability and the repair cost of all the structural and non-structural components and contents of the building. These methodologies were originally devised for a single building located at a specific site. However, they are also applied with little or no modifications in portfolio loss estimation to one or more archetypes representing an entire class of buildings that could be located anywhere within a region. This is an important observation that is central to the discussion below.
3.2 Vulnerability Functions for Single Buildings and for Building Portfolios: The Present
In practice, for portfolio loss estimation, for any building class in a given country an engineer either.
-
(a)
selects and adopts vulnerability functions available in the literature (perhaps based on data not from the same region of interest) often without an in-depth scrutiny, or
-
(b)
develops new numerical functions (FEMA 2003) using the global or the component-based approach but without paying enough attention to the ground motions used to estimate the response.
The former route should be followed with extreme caution. Even if two buildings in two different countries could be confidently categorized into the same class (e.g., low-rise ductile RC frame building), which is not always the case, their vulnerability functions would likely differ, sometimes considerably. Buildings in different parts of the world are, in fact, the final results of different design codes and construction practices. Hence, they naturally have different structural characteristics and seismic performance. Therefore, analysts usually develop region or country-specific vulnerability functions (Bal et al. 2008; Villar-Vega et al. 2017). Note that even in those cases where building codes are similar in two countries, the performance of two like buildings may still not be similar due to the different levels of code enforcement and construction quality assurance that may exist in the two countries. The custom of utilizing vulnerability functions from other countries is clearly a potential source of bias on the resulting loss estimates.
The latter route, which is clearly preferable, is not free of hurdles either. For many years, as alluded to earlier, it has been a common practice in the regional loss assessment to select one (or more) archetype building in a class and to assess its response using a set of ground motions. The set of recordings, which is typically selected without particular care to the local seismicity and level of hazard in the country, is utilized as input to perform Incremental Dynamic Analysis, IDA (Vamvatsikos and Cornell 2002) or some form of multi-stripe or cloud analysis (Jalayer 2003). The resulting vulnerability function is then applied to all the buildings in the class regardless of where they are located in the country.
However, the application of this methodology, originally intended for a given building at a given site, has shown that reliability of the resulting vulnerability function is achieved only when ground motions consistent with the hazard at the site are selected and when the ground motion IM utilized to predict the vulnerability is carefully chosen. This is a generally less understood but very important aspect. In simple words, the vulnerability function of a building does not only depend on the building itself but also on the characteristics of the ground motions that the building may experience in its lifetime. The reason for this dependence is hidden in the way vulnerability functions are constructed. Vulnerability functions are based on only one ground motion IM (almost always the first-mode spectral acceleration, SAT1, or the peak ground acceleration, PGA) leaving everything else unaccounted for. Given the same level of IM, however, the other characteristics of the ground motions, such as their spectral content and duration that do affect the response, are systematically different at different sites. They differ because they depend on the parameters (e.g., magnitude and distance of the building site from the main seismogenic sources) of the local earthquakes that most contribute to the hazard at a given site. In general, different sites have different controlling earthquakes that generate ground motions with different unaccounted-for characteristics that, in turn, cause vulnerability functions of identical buildings at different sites to be distinct.
Figure 11.4 shows the profiles of two commonly used building response measures for vulnerability assessment, the median inter-story drift ratio (IDR) and peak floor acceleration (PFA), of an identical 7-story building located in three different cities in Turkey: Istanbul, Ankara and Erzincan. The profiles shown for these three cities are the mean values obtained by running three different sets of ground motions with the same intensity of SAT1 = 0.35 g (Kohrangi et al. 2017b), but with spectral shapes consistent with the hazard of each one of the three sites. The hazard consistency was imposed via the Conditional Spectrum (CS) method (Jayaram et al. 2011; Kohrangi et al. 2017a), which along with the Generalized Conditional Intensity Measure, GCIM, (Bradley 2010) method form the state-of-the-art for performing site-specific hazard-consistent record selection. The profiles for both IDR and PFA at the three sites are clearly different, a difference that is lost in all regional portfolio loss assessment studies performed nowadays. As explained earlier, in practice, to develop vulnerability functions for archetype buildings, an engineer would take a readily available set of ground motions, such as the FEMA-P695 records, and carry on response analysis. The results of this exercise for the same 7-story building subject to the far-field record set of FEMA-P695 scaled to SAT1 = 0.35 g are also shown in Fig. 11.4. In this particular case, by chance, the FEMA-P695 records (all scaled to the same SAT1 = 0.35 g as done for the other three city-specific record sets) appear to generate more severe demands in the structure for both IDR and especially PFA than the other three sets. The larger damageability of this FEMA-P695 set holds also for IDR and PFA profiles computed for records scaled to other SAT1 levels. If this FEMA-P695 set of records were used to derive a vulnerability function for this archetype 7-story RC building and this function were used to estimate losses for all midrise RC buildings of that era in Turkey, the losses would be severely overestimated for all such buildings especially those in the two most heavily populated cities of Istanbul and Ankara. Any other random set of ground motions other than the FEMA-P695 would have generated different vulnerability functions with unknown reliability.
This simple exercise clearly supports the necessity of using ground motions that are site-hazard consistent and the consistency can be enforced for specific sites using CS, as we did, or GCIM. In a portfolio loss assessment, however, there are thousands of sites and developing one vulnerability function per site for each class of buildings would be highly impractical. The alternative question here is: “How can we make a ground motion record selection that is consistent with the seismic hazard in a region and not at a single site?” This selection would lead to a vulnerability function that is, on average, appropriate for all buildings in the region of interest.
3.3 Vulnerability Functions for Building Portfolios: The Future
The best practical strategy to tackle this problem is to develop vulnerability functions based on a ground motion IM that has the highest possible predictive power of the structural response engineering demand parameters (EDPs) that are used as building response metrics. This ideal IM would also need to be “predictable” given the usual parameters of the causative earthquake, such as magnitude and rupture mechanism, and of the site, such as local soil conditions and distance from the rupture. If this ideal IM were identified, an engineer would be able to predict with very little uncertainty the values of all the EDPs in a building caused by a ground motion with only the knowledge of the value of such an ideal IM. In the literature this ideal IM would be called perfectly “sufficient” (Luco and Cornell 2007). In this hypothetical case, ground motions from different regions with different durations and different causative magnitude earthquakes but with the same value of this ideal IM would generate the same distribution of building response. In simple terms, this ideal IM would make consistency with the site hazard in the ground motion record selection essentially irrelevant for building response assessment. In the context of portfolio analysis, the identification of this IM would remove the dependency of the vulnerability function from the selection of the site-specific ground motions, which is evident in Fig. 11.4 when SAT1 was used as the conditioning IM.
In the last decade, many studies (Cordova et al. 2000; Tothong and Luco 2007; Bianchini et al. 2010; Eads et al. 2015; Eads et al. 2013; Kazantzi et al. 2015; Vamvatsikos and Cornell 2005; Kohrangi et al. 2016a) focused on application of advanced IMs to reduce, to the extent possible, the dependency of the response and, therefore, of the vulnerability function on the site hazard. In particular, after the promising results in the assessment of site-specific building-specific losses (Kohrangi et al. 2017a), the authors proposed a multi-site record selection scheme for developing vulnerability functions for portfolio loss assessment that is both practical and reasonably accurate (Kohrangi et al. 2017b). This approach uses the average spectral acceleration, AvgSA, as an advanced IM for response prediction while a modification of the CS method to account for AvgSA at multiple sites is used to ensure the hazard consistency at the regional, rather than at the site, level. The regional hazard consistency is obtained by the law of total variance, which incorporates the impact of multiple sites into a single record set. The weight associated to each site, for example, was chosen to be proportional to the replacement cost of that building class at that site as a fraction of the total replacement cost of that class in the entire country. This method provides a unique set of ground motion records that has a balanced contribution from the ground motions relevant to the hazard at the different sites to use as input to the vulnerability analyses computations. Note that the amount of response analyses that the engineer needs to carry out is identical to before, only the records are selected more judiciously.
Figure 11.5b and d show the results of this AvgSA-based multi-site-hazard-consistent approach for our illustrative example entailing the same 7-story reinforced concrete building located at the three sites of Istanbul, Ankara and Erzincan. More specifically, Fig. 11.5b shows a comparison of collapse fragility curves and Fig. 11.5d displays the vulnerability functions obtained using ground motions records selected using the site-specific approach for the three cities, the proposed multi-site AvgSA-based approach discussed above, and the FEMA-P695 set (this last one scaled as done in an IDA framework to multiple IM levels). The multi-site fragility and vulnerability functions are, for all practical purposes, almost indistinguishable from those developed specifically for the three cities. This means that utilizing this approach to develop fragility and vulnerability functions can potentially remove the existing bias from portfolio loss estimates. It is also apparent that the use of a much more sufficient IM, such as AvgSA, makes the family of fragility curves to be very tight regardless of the method used to select the input ground motions. Figure 11.5a and c show the fragility and vulnerability functions, respectively, computed using the same multi-site-hazard-consistent methodology of Kohrangi et al. (2017b) but this time based on the poorly “sufficient” SAT1, as done routinely in practice, rather than on AvgSA. The families of curves are much more dispersed and the proposed multi-site approach is less efficient in reducing the bias that may stem from using randomly or, in any case, poorly selected ground motion records.

Adopted from Kohrangi et al. (2017b)
Collapse fragility curves (top panels) and vulnerability functions (bottom panels) of a 7-story reinforced concrete frame building located at three sites in Turkey using two different conditioning IMs: SAT1 in (a) and (c); AvgSA in (b) and (d).
The effects on the risk estimates of the IM chosen for developing vulnerability functions and of the record selection scheme selected for the computations of the vulnerability functions are shown in Fig. 11.6. This figure shows the different estimates of the mean annual rate of exceedance loss curves for the 7-story reinforced concrete building discussed so far located in the three cities of Ankara, Erzincan, and Istanbul. These curves were computed by convolving the hazard curves for soft soil conditions for these three cities with the corresponding vulnerability curves in Fig. 11.5c and d. Different important observations stem from the inspection of Fig. 11.6. The first one is certainly the difference in the site-specific loss exceedance curves for the same city obtained using SAT1 and AvgSA as the pivotal IM. The difference stems from the lack of sufficiency especially of SAT1 and, to a lesser extent, of AvgSA but, as argued in Kohrangi et al. (2017a), the AvgSA-based curves are statistically more robust and closer to the true but unknown curve. Therefore, for the discussion at hand, the benchmark curve for the building in each city should be considered the site-specific curve based on AvgSA. The second observation is on the variability between the loss exceedance curves obtained for the same city using the different approaches. This variability is much larger for the family of curves based on SAT1 than for that based on AvgSA. Selecting a set of records for vulnerability computations that is not consistent with the site and regional hazard, such as the FEMA-P696 set for example, can lead to severe bias in the risk estimates. In the case at hand, by chance that record set would have provided reasonably accurate results only for Erzincan but severe overestimation of the risk for the much more important cities of Istanbul and Ankara. All these differences, however, almost vanish when AvgSA is used for the development of vulnerability functions. Because of the much higher sufficiency of this IM, even the selection of the records used for developing vulnerability curves becomes much less important. The estimates of the loss exceedance curves are closely clustered around the benchmark regardless of the ground motion records selection scheme adopted.
3.4 Final Remarks
To conclude, the current practice of developing vulnerability functions via the analytical method for classes of buildings is the most widely used in commercial models for portfolio loss estimation. This practice, which hinges on using inferior and insufficient IMs, such as SAT1, to predict structural responses coupled with a primitive ground motion record selection is prone to introducing bias of unknown amount and sign in the final loss estimates. The use of AvgSA, which is a significantly more sufficient IM, coupled with a careful record selection that balances the characteristics of ground motions at the sites of interest in the study region is surfacing as a much more promising approach for removing such a bias. As an intermediate step, with limited effort the current practice could embrace the use of the SAT1-based multi-site-hazard-consistent approach, which by virtue of producing vulnerability functions that are balanced in terms of ground motion characteristics likely expected at the different sites of the study region, may at least reduce, if not eliminate, the bias in the loss estimates.
4 Beyond Ergodic Seismic Hazard Estimates and Impact on Risk
A core component of probabilistic seismic hazard is the ground-motion prediction equation (GMPE). For any prospective earthquake, in the second part of the hazard module of any earthquake risk assessment model a GMPE is required to predict the distribution of any (log) ground-motion intensity measure, IM, at any site of interest in the affected region. The (log) IM at a given site is modeled as a Gaussian distribution Ɲ(μ, σ), where μ is the median value of the IM and σ is the standard deviation given the parameters of the earthquake, typically the earthquake moment magnitude (Mw), the distance of the rupture from the site (e.g., the Joyner-Boore metric RJB), and the site conditions (often described by the time averaged shear-wave velocity Vs30 in the top 30 m). However, to obtain empirical GMPE that yield reliable and stable IM median predictions even for scenarios beyond those ever recorded at a site, the developers apply statistical techniques on datasets of ground-motion observations from a variety of earthquakes recorded at a multitude of sites scattered across the globe. This practice, which is dictated solely by the scarcity of data at any single site, essentially substitutes long-term sampling of ground motions at a given site with short-term sampling at several other sites with similar conditions. In statistical parlance, this is the so-called ergodic assumption in GMPEs (Anderson and Brune 1999). The ergodic assumption predicates that the generic μ of a GMPE predicts theoretically and physically constrained ground motions over a wide range of magnitude, distance, and site conditions. In other words, ground motions from earthquakes occurred somewhere else in the world and recorded at sites similar to the one considered are acceptable proxies for the ground motions that local earthquakes could cause at the specific site of interest. In this ergodic framework, all the spatiotemporal variabilities of the geophysical properties unaccounted in the ground-motion prediction are considered natural randomness, and are relegated to the aleatory variability σ of the IM. These geophysical elements of a seismic process can be broadly identified as those related to the physics of earthquake ruptures, shear-wave propagation paths, and receiving sites’ conditions.
Recent years have witnessed an exponential growth of ground-motion observations from several seismically active regions of the world. GMPEs have benefitted tremendously from these high-quality datasets, especially in reducing the epistemic (modelling) uncertainty of μ at a given site for a wide variety of scenarios. However, in a review of the 50 years of GMPE development, Douglas (2014) reported a counter-intuitive increase in σ, namely an increase in the unresolved spatiotemporal variability of seismic processes. In a probabilistic seismic hazard assessment framework, an increasing σ implies an increasing likelihood of rare (and large) ground motions from even moderate-sized earthquakes (Bommer and Abrahamson 2006). In turn, this implies the paradox that, despite an increasing amount of high-quality ground-motion data, the ergodic hazard estimates become more uncertain and, in turn, the associated likelihood of observing large damage and losses due to earthquakes increases. To mitigate the negative consequences of this issue, GMPE developers have started opting out of the ergodic assumption to focus on explaining some of the apparent natural randomness of (geophysics of) seismic processes. In other words, the shift towards non-ergodic GMPEs has started.
The ergodic assumption can be relaxed with the discovery of repeatable seismic phenomena in the data and in explicitly modelling them into the μ of the GMPE, as discussed in the next section. This operation changes the median estimate from the value of the ergodic one and, at the same time, reduces the aleatory variability σ. Figure 11.7 is an illustration of shifting from a generic ergodic prediction Ɲ(μ, σ) to three non-ergodic level i specific predictions with their own unique distributions Ɲ(μi, σi); where levels i = 1, 2, 3 could be ruptures, paths, sites, or any spatiotemporal-specific (characteristic). Plainly put, the more non-ergodic a GMPE becomes, the more spatio-temporally specific its μi, and lower its σi will become.
Illustrative example of the distribution of an IM from a scenario event at four sites with identical Vs30 and distance from the causative earthquake. The effects on the IM distribution in the three non-ergodic cases due to the reduction in σ and shift in μ is evident. Courtesy: Dr. Norman Abrahamson
4.1 Partially Non-ergodic GMPEs
In the quest for non-ergodic GMPEs, it is first necessary to identify the three distinct pieces that contribute to shaping the ground motion features recorded at any given site, namely.
-
1.
earthquake ruptures and their seismogenic sources,
-
2.
shear-wave propagation paths and their regions, and
-
3.
receiving sites’ geology and topography.
For appropriately modeling them, the second step involves the characterization of their spatiotemporal specifics, as discussed below.
Although the interaction between these components is complex, the pursuit for non-ergodic GMPEs has been quite rewarding in the past few years. Anticipating fully non-ergodic GMPEs, Al Atik et al. (2010) proposed a taxonomy for the components of σ (McGuire 2004), which was complemented by several follow-up studies leading up to the most recent one by Baltay et al. (2017).
4.1.1 Characterization of Earthquake Ruptures and Their Seismogenic Sources
Among the various parameters characterizing an earthquake rupture, most GMPEs use only the Mw as a predictor variable in the functional form for estimating μ of the ground-motion IM at a site. However, Mw is only indicative of the size of an earthquake rupture, and not as much of the amount of shear-wave energy radiated from the elastic rebound, which is the actual cause of ground-motion at a site. This radiated energy is best correlated to the tectonic stress released by a single rupture, and is (generally) quantified as stress-drop in the rupture’s Brune (1970) shear-wave spectrum.
Depending on their spatial and temporal origins, earthquake ruptures of identical Mw may show very dissimilar stress-drops, e.g., see Cotton et al. (2013). This may arise from the differences in the tectonic regimes, crustal deformation rates, periodicity of stress release, and other tectonic processes that lead to a rupture. For example, a recent study by Bindi and Kotha (2020) showed that the M6.5 Friuli earthquake (1976) had substantially higher stress-drop than the recent M6.5 Norcia earthquake (2016), which in-turn, released higher energy than the more recent and nearby M6.3 L’Aquila earthquake (2009). Therefore, the functional form for predicting μ of a non-ergodic rupture-specific GMPE would use at least the rupture-specific stress-drop as a predictor variable. This enhancement leads to a substantially lower value of the non-ergodic σ (Bindi et al. 2018b, 2019).
In practice, however, the stress-drop of even the largest ruptures is hard to predict. Therefore, a reasonable level of non-ergodic source-specificity can be achieved by spatially localizing several ruptures and their stress-drops to seismogenic sources; such as fault systems, hypocentral depths (Abrahamson et al. 2014), tectonic localities (Kotha et al. 2020), epicentral coordinates (Landwehr et al. 2016), or epicentral neighborhood (Lin et al. 2011). For example, Fig. 11.8 is a result from the recently developed partially non-ergodic GMPE of Kotha et al. (2020) from the Engineering Strong Motion dataset (Lanzano et al. 2018; Bindi et al. 2018a). In their study, Kotha et al. (2020) grouped the 927 shallow crustal ruptures of 3.0 < Mw ≤ 7.4 occurred between 1976–2016 in the pan-European region into tectonic localities (groups of seismic sources) devised in the purview of European Seismic Hazard Model 2020 (ESHM20). These localities are larger regions containing tectonically similar seismic sources. In developing the locality-specific GMPE, the systematic differences between the ground-motion IMs caused by earthquakes occurred in various localities are quantified into a quantity called δL2Ll, where l indexes one of the locality polygons shown in Fig. 11.8. Ruptures originated in the red localities have produced, on average, stronger ground motions than those occurred in the blue localities. With respect to the ergodic median of the GMPE, the median IM of the former ground motions was higher than the ergodic median (like Non-Ergodic Case 2 in Fig. 11.7) and vice versa for the latter (like Non-Ergodic Case 3 in Fig. 11.7). Such estimates, if assumed temporally stationary as customarily done, can be used to predict partially non-ergodic locality-specific ground-motion IMs for prospective ruptures.
Map illustrating the spatial variability of locality-specific ground-motion prediction adjustments to the ergodic median IM, here PGA. Red polygons are tectonic localities generating earthquake ruptures that produce a median PGA higher than the ergodic median, while blue polygons correspond to those producing a median PGA lower than the ergodic median, as estimated from the Engineering Strong Motion dataset by Kotha et al. (2020)
Geologists and geophysicists have recently invested considerable energy in identifying parameters that can help characterize the spatiotemporal variability of seismogenic source properties (see the localities in Fig. 11.8), such as fault maturity (Radiguet et al. 2009), seismic moment-rate density (Weatherill et al. 2016), and rupture velocity (Chounet et al. 2018). On the other hand, the temporal variability of rupture characteristics has proven more difficult to resolve empirically, because it can be associated to the characteristics of the propagation medium (e.g., crustal velocity structure), or to the characteristics of the source properties (e.g., fault healing), or to some combination of both. Nevertheless, assimilation of large amounts of ground-motion data from active seismic sources helps resolving at least the spatial variability, while assuming temporal stationarity for now.
4.1.2 Characterization of the Shear-Wave Propagation Paths and Their Regions
While source-specific, in lieu of rupture-specific, predictions are still in development, the investigation of the spatial variability of shear-wave propagation effects has seen a much earlier start, e.g. Douglas (2004). Ergodic GMPEs typically predict ground-motions that decay identically with distance towards the site regardless of the region of the world where they are applied. Several GMPEs have, however, already quantified the regional differences in attenuation of ground-motion with distance. The lithospheric properties, such as shear-wave velocity and depth to Moho, vary rapidly across the seismically active regions. To account for these variations, for instance, Kotha et al. (2016) proposed a partially non-ergodic region-specific GMPE for the pan-European regions, where a region encompasses several possible paths of shear-waves. The region-specific μ of that GMPE distinguishes the much faster decay (with distance) of high-frequency ground-motion IMs in Italy compared to that in the rest of Europe. Similarly, the GMPE by Kale et al. (2015) quantified regional differences in attenuation between Iran and Turkey; while Boore et al. (2014), Campbell and Bozorgnia (2014), and Abrahamson et al. (2014) models distinguish attenuation between California, Japan, New Zealand, China, and other active regions.
More recently, using the 18,222 records in the ESM dataset,Footnote 2 Kotha et al. (2020) quantified the regional differences in apparent anelastic attenuation of IMs across 46 regions of the TSUMAPS-NEAM regionalization model (Basili et al. 2019) spanning the most seismically active regions of pan-Europe. The systematic differences across regions in the anelastic attenuation are quantified in the quantity δc3,r mapped in Fig. 11.9. With respect to the median of high-frequency IM of the ergodic GMPE, shear-waves traversing the blue regions in Fig. 11.9 experience a faster anelastic decay (i.e., lower median), while those traversing the red regions propagate more efficiently (i.e., higher median).
Map illustrating the spatial variability of region-specific ground-motion adjustments to the ergodic median IM (here PGA). Red polygons are regions with weaker than average anelastic attenuation of PGA (i.e., higher median PGA), while blue polygons correspond to regions with anelastic attenuation of PGA stronger than global average (i.e., lower median PGA), as estimated from the Engineering Strong Motion dataset by Kotha et al. (2020)
4.1.3 Characterization of Receiving Sites’ Geology and Topography
The momentous shift towards the development of non-ergodic GMPEs has been propelled by the need of resolving the third piece of the puzzle, namely the site-to-site variability of ground-motion characteristics. Anderson and Brune (1999) triggered the need to resolve ergodicity of GMPEs by first identifying the epistemic and aleatory components of ground-motion variability. Assuming a specific site’s response to seismic action is temporally invariable (i.e., site’s local soil and topographic conditions do not change dramatically between two consecutive earthquakes), a sufficient number of recordings at a given site would allow quantifying the site-specific response with reasonably low epistemic uncertainty. This uncertainty would also decrease asymptotically with more recordings. At such sites with multiple recordings, the aleatory site-to-site response variability, of course, does not apply anymore and during ground motion prediction it can be removed from the ergodic σ of the GMPE. This reduced non-ergodic σ, sometimes called the single-site σ in the literature, can be 30 to 40% smaller (Rodriguez‐Marek et al. 2013) than its ergodic counterpart.
In addition to the source (Fig. 11.9) and path (Fig. 11.8) specific adjustments to ergodic GMPEs, the increase in high quality ground motion data has also allowed developing site-specific adjustments for many locations with multiple recordings. For example, Kotha et al. (2020) compiled site-specific (Fig. 11.10) adjustments for 1,829 sites in the ESM dataset, out of which 1,047 have recorded more than 3 earthquakes. In this figure, the locations of sites with red color are those at which the median of the recorded PGA values was systematically higher than the generic median of the ergodic GMPE for the same scenario earthquakes. The opposite trend applies to the blue marked locations. The development of such non-ergodic site-specific GMPEs and their application in seismic hazard and risk assessment are elaborated in a few recent studies (Kotha et al. 2017; Faccioli et al. 2015; Rodriguez‐Marek et al. 2013), where the quantified differences between ergodic and non-ergodic site-specific assessments are shown to be enormous.
Map illustrating the spatial variability of site-specific ground-motion adjustments. Red markers are sites whose median of the recorded PGA values for past earthquakes was larger than the ergodic median PGA. The opposite holds for the median PGA at the blue marker locations. The adjustments, expressed in terms of the δS2Ss quantity mapped here, were estimated from the Engineering Strong Motion dataset by Kotha et al. (2020)
4.2 Effects of Partially Non-ergodic GMPEs on Risk Estimates
Following the development of partially non-ergodic GMPEs, the seismic hazard assessment community has started adopting these into practical applications (Weatherill et al. 2020; Stewart et al. 2017b; Walling 2009). A first attempt in assessing the impact on risk estimates due to shifting from ergodic to more accurate partially non-ergodic GMPEs adjusted for site-specific ground-motion predictions was done by Kohrangi et al. (2020) for single structures located at three sites in Turkey. This study considered elastic-perfectly plastic SDOF systems with initial elastic periods of T1 = 0.2 s, T1 = 0.5 s and T1 = 1.0 representing ductile moment-resisting-frame buildings with different low-to-medium heights. The three sites (Table 11.1) were chosen based on their Vs30 values reported in the RESORCE dataset (Akkar et al. 2014) to represent rock sites (Site #1), very stiff sites (Site #2), and stiff sites (Site #3) as classified in the Eurocode 8. Table 11.1 lists also the number of ground-motion recordings used in estimating their site-specific adjustments, i.e. their site-specific δS2Ss values as done for the sites in Fig. 11.10.
Kohrangi et al. (2020) compared the hazard and risk estimates at these three sites obtained using the area source seismicity model of SHARE (Woessner et al. 2015) and the two versions of the Kotha et al. (2016) GMPE. The ergodic version relies on the sites’ Vs30 (a proxy of soil stiffness) to predict soil-specific (but site-generic) ground motions, while the non-ergodic version ignores Vs30 and uses the site-specific empirical amplification factors δS2Ss to predict site-specific ground motions. The ergodic (dotted) and non-ergodic (solid) hazard curves at the three sites, are compared in Fig. 11.11. It is evident that the differences between ergodic and non-ergodic hazard estimates are site-dependent, period-dependent, and hazard-level-dependent. For instance, the site-specific hazard curves at rock Site #1 are significantly below the ergodic estimates, a trend that reflects the stronger local deamplification of short and long period ground motions at Site #1 compared to other sites (in the dataset) with similar Vs30. Meanwhile, at the stiff soil Site #3, moderate period (T1 = 1.0 s) ergodic and non-ergodic hazard curves are almost identical, but differ significantly at T1 = 0.5 s.
Comparison of SA at T1 = 0.2, 0.5, 1.0 s (left to right columns) ergodic (dotted) and non-ergodic site-specific (solid) hazard curves (top-row) and ductility exceedance risk curves (bottom-row) for identical buildings at the three sites (color coded). Courtesy: Kohrangi et al. (2020)
The results of the response analyses carried out on these three structures using site-specific hazard consistent sets of ground motion records led to the vulnerability curves shown in Fig. 11.12. The risk estimates for these three structures at the three sites displayed in the form of loss exceedance curves in Fig. 11.13 were obtained via convolution of these vulnerability curves and the hazard curves shown in Fig. 11.11. The impact due to the more accurate site-specific non-ergodic GMPEs is evident. In general, the traditional ergodic GMPE would lead to a severe underestimation of the risk for all three structures at Site #3 but to a lesser extent for the more flexible one than for the stiffer one. Vice versa for Site #1 where the ergodic GMPE would lead to an overestimation of the risk. At Site #2 the ergodic GMPE yields very similar but slightly conservative risk estimates compared to the more precise estimates obtained via the site-specific non-ergodic GMPE.
Hence, as concluded by Kohrangi et al. (2020), this example shows that the traditional approach may lead to biased risk estimates whose amplitude and sign are impossible to predict a priori unless high quality site-specific ground-motion data (Bard et al. 2019) allow the development of site-specific non-ergodic GMPEs. Based on these results, the use of the non-ergodic approach is recommended, whenever existing data allow it. However, further advancements of non-ergodic GMPEs are necessary before being routinely utilized in real life risk assessment applications.
5 Sources of Bias in Pricing of Earthquake Insurance Policies
As mentioned earlier, one of the key decisions that hinges on the results of catastrophe risk models is the determination by insurance and reinsurance companies of how much premium is fair to charge to cover the cost of an insurance product and generate sufficient profit. In insurance parlance this activity is called pricing. The price that a customer pays for the product, namely the market price, is a function of the so-called technical price, which can be either higher or lower of the market price depending on internal business strategies of the company. In turn, the technical price consists of:
-
1.
The pure premium, which is the expected loss that the insurer can expect to pay, on average, every year over a long period of time. This quantity, is simply the model-based estimate of the Average Annual Loss (AAL) mentioned in the introduction.
-
2.
Expense loading (included to account for internal operational costs, taxes, fees, commissions, reinsurance and retrocession costs, cost of capital, etc.)
-
3.
Profit loading
-
4.
Risk loading (to account for unmodeled perils and unknowns)
In this section we discuss the main contribution to the technical price, namely the pure premium, which is the only part of a technical price that has a scientific basis Figs. 11.14, 11.15 and 11.16 compare the AALs for the structures considered in the examples discussed in the three main sections of this chapter. These AALs were obtained using both the traditional approach and the enhanced approach suggested in those sections.
AAL ratio for the entire building inventory of the Umbria region (Section 11.2) (as a percentage of the total replacement cost) computed using mainshock only seismicity and all seismicity for two distinct periods
Estimates of the AAL ratio for the same 7-story reinforced concrete building, whose vulnerability functions were derived based on SAT1 (left) and AvgSA (right) (Section 11.3). In blue the estimates obtained using site-hazard-consistent ground motion selection; in red the estimates computed using the regional-hazard-consistent ground motion selection proposed by Kohrangi et al. (2017b); in yellow the estimates from the application of the FEMA-P695 ground motion record set
Estimates of the AAL ratio for the three structures with the fundamental period of vibration of T1 = 0.2, 0.5, 1.0 s located at the three sites (Section 11.4). In blue the estimates based on the site hazard computed via a site-specific non-ergodic GMPE; in red the estimates based on the site hazard computed via a traditional ergodic GMPE
More specifically, Fig. 11.14 shows the AAL estimates for the active and random seismicity years computed for the building stock of Umbria using mainshock only seismicity (i.e., the traditional approach) and clustered seismicity that includes all other events. Not unexpectedly given the different loss exceedance probability curves shown in Fig. 11.3, the AAL ratio for the active year starting on 26/04/2017, (i.e., just after the tail of the 2016–2017 Central Italy sequence) was found to be three times higher than that for a random year and about four times higher compared to the estimate obtained using a conventional mainshock-only seismicity model. If the onset of the investigation time for the active period were moved to the middle of the 2016–2017 Central Italy sequence, the AAL estimates would have been a much higher multiple of the mainshock-only 3.4 per mille estimates of Fig. 11.14. Note that these AAL values are for the aggregated building inventory of the entire Umbria. Papadopoulos and Bazzurro (2021) pointed out, however, that this AAL increase due to the clustered seismicity is highly dependent on the vicinity of each building to the epicenters of the then-ongoing sequence. In fact, individual building AAL estimates were found amplified by more than one order of magnitude (compared to the Poissonian mainshock-only case) close to the epicenter of the 2016 Norcia earthquake, while they converge to the estimates for a random year as one moves away from it.
Figure 11.15 shows the estimates of the AAL ratio for the same 7-story reinforced concrete building located at sites in Ankara, Istanbul and Erzincan using SAT1 (left) and AvgSA (right) as the IM adopted for the development of the vulnerability function. The same considerations made for the mean annual rate of exceedance loss curves in the corresponding section hold here as well. The blue bars on the right panel of Fig. 11.15 computed using site-specific hazard-consistent ground motions and AvgSA, a much superior and more sufficient IM, are to be considered as the closest estimates to the true but unknown values of the AAL. The traditional approach that entails developing vulnerability functions using SAT1 as IM and sets of ground motions not necessarily consistent with the regional hazard is prone to causing bias of unknown sign (here positive, namely too conservative) and amplitude in the AAL estimates. If one wants for historical reasons to keep SAT1 as the IM of choice, then the multi-site approach of Kohrangi et al. 2017b for selecting regionally hazard consistent ground motions is a practical procedure. The recommendation, however, as it appears clearly from an inspection of the right panel of Fig. 11.15, is to use that procedure anchored to AvgSA instead. It is remarkable, however, how the use of AvgSA decreases the importance of record selection. Even the random set of ground motions provided by FEMA-P695 yields excellent AAL estimates, at least for this example.
Finally, Fig. 11.16 displays the estimates of the AAL ratio for the three structures with the fundamental period of vibration of T1 = 0.2, 0.5, 1.0 s located at the three sites for which partially non-ergodic GMPEs were developed. It is clear that only for Site #2 the estimates obtained using the traditional approach whose hazard is computed via ergodic GMPEs (red bars) are similar to the more precise ones that account for the specificity of the site for ground motion prediction. This occurs because the non-ergodic GMPEs and the ergodic GMPEs have similar median values for spectral accelerations in the neighborhoods of T1 of the different structures. The characteristics of Sites #1 and #3 are significantly different than those of the average sites with same Vs30 whose recordings were used to develop the ergodic GMPE. Therefore, the traditional approach would overestimate by about 100% the AAL for all structures at Site #1 and underestimate the AAL at Site #3 by 40% for the T1 = 1.0 s structure and by more than 100% the AAL of the two stiffer structures. Unfortunately, how much the peculiarities of each site deviate from those of the average site with same Vs30 is a piece of information seldom known a priori. Therefore, unless more data (enough recordings of past earthquakes in this case) at more sites becomes available the widespread use of more precise non-ergodic GMPEs will be limited to assessing hazard and risk at specific sites and its use in portfolio risk analysis will be prevented.
6 Conclusions and Recommandations
Catastrophe risk assessment models are at the core of many risk mitigation decisions made by a wide variety of stakeholders. The quality of these models and, consequently, the accuracy of the risk estimates they provide have steadily improved in the last 30 years since the first ones were developed. However, the current models used for commercial portfolio risk analyses have some known caveats that may lead to biased risk estimates. In this chapter we discussed two shortcomings existing in all current earthquake risk assessment models, namely the neglect of earthquake sequences and the use of region-generic ground motions for developing vulnerability functions.
Ignoring the spatial clustering of seismicity can induce a negative bias (i.e., one that leads to unconservative values) in loss estimates in a few different ways. Naturally, accounting for the earthquakes that are normally discarded during declustering yields higher AAL estimates. The AAL increase can arguably depend on several factors such as the seismicity conditions prior to the time when the analysis is conducted, the degree of seismicity clustering in a given region, the susceptibility of the building stock to damage accumulation, and the size and spatial distribution of the portfolio, to name a few. As an illustrative example, we presented the work of Papadopoulos and Bazzurro (2021), which compared AAL estimates for the Umbria region in Central Italy using different initial conditions, i.e. an active 1-year period as described earlier and a year with random initial conditions. The underestimation of the AAL, especially in periods of heightened seismicity, for all assets close to ongoing sequences is just too large to ignore.
Using insufficient ground motion IMs, such as SAT1, and hazard-inconsistent ground motions for developing analytical vulnerability functions for classes of like-buildings are two features of the current practice that should be discontinued. The resulting vulnerability curves could be unbiased either positively or negative to such an extent unknown to the structural engineers who developed it. Practical workaround techniques that address both issues have been shown here to work well in removing most of such a bias. Their applicability in real life portfolio risk assessment is certainly recommended. Of the two suggested techniques, the use of AvgSA as the pivotal IM for vulnerability functions development is the one that we expect to be adopted sooner, since it is both easier to grasp for engineers and the most effective of the two.
The use of non-ergodic GMPEs is certainly one of the future enhancements of earthquake risk assessment models for portfolios of structures. However, the road to arrive to destination still requires collecting more data and doing more research before its use can be recommended for this purpose. It must be noted, though, that partially non-ergodic GMPEs that account for specific characteristics of both seismogenic sources and seismic wave traveling paths and attenuation in certain regions are certainly closer to practical fruition. In all the regions where enough data is available, the use of partially non-ergodic GMPEs for hazard and risk assessment should certainly be considered.
References
Abrahamson NA, Silva WJ, Kamai R (2014) Summary of the ASK14 ground motion relation for active crustal regions. Earthq Spect 30:1025–1055
Akkar S, Sandıkkaya M, Şenyurt M, Sisi AA, Ay B, Traversa P, Douglas J, Cotton F, Luzi L, Hernandez B, Godey S (2014) Reference database for seismic ground-motion in Europe (RESORCE). Bull Earthq Eng 12:311–339
Akkar S, Sucuoglu H, Yakut A (2005) Displacement-based fragility functions for low- and mid-rise ordinary concrete buildings. Earthq Spect 21:901–927
Atik AL, Abrahamson N, Bommer JJ, Scherbaum F, Cotton F, Kuehn N (2010) The variability of ground-motion prediction models and its components. Seism Res Letters 81:794–801
Anderson JG, Brune JN (1999) Probabilistic seismic hazard analysis without the ergodic assumption. Seism Res Letters 70:19–28
ATC-13 (1985) Earthquake damage evaluation data for California. In: ATC-13, R. (ed.) Applied Technology Council. Redwood City, California, USA
Bal İE, Crowley H, Pinho R, Gülay FG (2008) Detailed assessment of structural characteristics of Turkish RC building stock for loss assessment models. Soil Dyn Earthq Eng 28:914–932
Baltay AS, Hanks TC, Abrahamson NA (2017) Uncertainty, variability, and earthquake physics in ground-motion prediction equations. Bull Seism Soc Am 107:1754–1772
Barbat A, Yépez Moya F, Canas J (1996) Damage scenarios simulation for seismic risk assessment in urban zones. Earthq Spect 12:371–394
Bard P-Y, Bora SS, Hollender F, Laurendeau A, Traversa P (2019) Are the Standard V S-Kappa host-to-target adjustments the only way to get consistent hard-rock ground motion prediction? Pure App Geoph, 1–20
Basili R, Brizuela B, Herrero A, Iqbal S, Lorito S, Maesano FE, Murphy S, Perfetti P, Romano F, Scala A (2019) NEAMTHM18 Documentation: the making of the TSUMAPS-NEAM tsunami hazard model 2018
Bianchini M, Diotallevi P, Baker J (2010) Prediction of inelastic structural response using an average of spectral accelerations. 10th International Conference on Structural Safety and Reliability (ICOSSAR09). Osaka, Japan
Bindi D, Kotha S-R, Weatherill G, Lanzano G, Luzi L, Cotton F (2018a) The pan-European engineering strong motion (ESM) flatfile: consistency check via residual analysis. Bull Earthq Eng, 1–20
Bindi D, Spallarossa D, Picozzi M, Scafidi D, Cotton F (2018b) Impact of magnitude selection on aleatory variability associated with ground-motion prediction equations: Part I—Local, energy, and moment magnitude calibration and stress-drop variability in central Italy. Bull Seism Soc Am 108:1427–1442
Bindi D, Kotha S (2020) Spectral decomposition of the engineering strong motion (ESM) flat file: regional attenuation, source scaling and Arias stress drop. Bull Earthq Eng, 1–26
Bindi D, Picozzi M, Spallarossa D, Cotton F, Kotha SR (2019) Impact of magnitude selection on aleatory variability associated with ground-motion prediction equations: part II—analysis of the between-event distribution in Central Italy. Bull Seism Soc Am 109:251–262
Bommer JJ, Crowley H (2006) The Influence of Ground-Motion Variability in Earthquake Loss Modelling. Bull Earthq Eng Des 4:231–248
Bommer JJ, Abrahamson NA (2006) Why do modern probabilistic seismic-hazard analyses often lead to increased hazard estimates? Bull Seism Soc Ame 96:1967–1977
Boore DM, Stewart JP, Seyhan E, Atkinson GM (2014) NGA-West2 equations for predicting PGA, PGV, and 5% damped PSA for shallow crustal earthquakes. Earthq Spect 30:1057–1085
Bradley BA (2010) A generalized conditional intensity measure approach and holistic ground-motion selection. Earthq Eng Struct Dyn 39:1321–1342
Brune JN (1970) Tectonic stress and the spectra of seismic shear waves from earthquakes. J Geoph Res 75:4997–5009
Brzev S, Scawthorn A, Charleson L, Allen M, Greene K, Jaiswal K, Silva V (2013) GEM Technical Report 2013–02. In: 2.0, G. B. T. V. (ed.). GEM Foundation, Pavia, Italy
Calvi G, Pinho R (2004) LESSLOSS. A European integrated project on risk mitigation for earthquakes and landslides. In: 2004/02, R. (ed.). European School for Advanced Studies in Reduction of Seismic Risk (ROSE School): Pavia, Italy
Calvi G, Pinho R, Magenes G, Bommer JJ, Restrepo-Vélez L, Crowley H (2006) Development of seismic vulnerability assessment methodologies over the past 30 years. ISET J Earthq Tech 43:75–104
Campbell KW, Bozorgnia Y (2014) NGA-West2 Ground motion model for the average horizontal components of PGA, PGV, and 5% damped linear acceleration response spectra. Earthq Spect 30:1087–1115
Chounet A, Vallée M, Causse M, Courboulex F (2018) Global catalog of earthquake rupture velocities shows anticorrelation between stress drop and rupture velocity. Tectonophysics 733:148–158
Cordova P, Deierlein G, Mehanny S, Cornell C (2000) Development of a two-parameter seismic intensity measure and probabilistic assessment procedure. In: The Second US-Japan Workshop on Performance-Based Earthquake Engineering Methodology for Reinforced Concrete Building Structures, September, 187–206
Cotton F, Archuleta R, Causse M (2013) What is sigma of the stress drop? Seismol Res Lett 84:42–48
D’ayala D, Meslem A, Vamvatsikos D, Porter K, Rossetto T (2014) Guidelines for analytical vulnerability assessment of low/mid-rise buildings. GEM Technical Report 2014–12: Global Earthquake Model Foundation, Pavia, Italy
Di Pasquale G, Orsini G, Romeo R (2005) New developments in seismic risk assessment in Italy. Bull Earthq Eng 3:101–128
Douglas J (2004) An investigation of analysis of variance as a tool for exploring regional differences in strong ground motions. J Seism 8:485–496
Douglas J (2014) Fifty years of ground-motion models. In: Second European Conference on Earthquake Engineering and Seismology (2ECEES): a joint event of the 15th European Conference on Earthquake engineering & 34th General Assembly of the European Seismological Commission
Eads L, Miranda E, Krawinkler H, Lignos DG (2013) An efficient method for estimating the collapse risk of structures in seismic regions. Earthq Eng Struct Dyn 42:25–41
Eads L, Miranda E, Lignos D (2015) Average spectral acceleration as an intensity measure for collapse risk assessment. Earthq Eng Struct Dyn
Faccioli E, Paolucci R, Vanini M (2015) Evaluation of probabilistic site-specific seismic-hazard methods and associated uncertainties, with applications in the Po Plain, northern Italy. Bull Seism Soc Am 105:2787–2807
FEMA-P58 (2012) Federal emergency management agency: Seismic performance assessment of buildings, prepared by the Applied Technology Council for the Federal Emergency Management Agency. In: AGENCY, F. E. M. (ed.). Washington, DC
FEMA-P695 (2009) Quantification of building seismic performance factors. 201 Redwood Shores Parkway, Suite 240, Redwood City, California 94065 Applied Technology Council
FEMA (2003) HAZUS-MH Technical Manual. In: AGENCY, F. E. M. (ed.). Washington, DC, USA
Field E, Porter K, Milner K (2017) A prototype operational earthquake loss model for California based on UCERF3-ETAS—A first look at valuation. Earthq Spect, 33
Gardner J, Knopoff L (1974) Is the sequence of earthquakes in southern California, with aftershocks removed, Poissonian. Bull Seism Soc Am 64:1363–1367
Haselton C, Baker J, Liel A, Deierlein G (2011) Accounting for ground-motion spectral shape characteristics in structural collapse assessment through an adjustment for epsilon. J Struct Eng 137:332–344
Jalayer F (2003) Direct probabilistic seismic analysis: implementing non-linear dynamic assessment. PhD Thesis, Stanford University
Jalayer F, Ebrahimian H (2016) Seismic risk assessment considering cumulative damage due to aftershocks. Earthq Eng Struct Dyn
Jayaram N, Lin T, Baker J (2011) A computationally efficient ground-motion selection algorithm for matching a target response spectrum mean and variance. Earthq Spect 27:797–815
Jayaram N, Shome N, Rahnama M (2012) Development of earthquake vunerability functions for tall buildings. Earthq Eng Struct Dyn 41:1495–1514
Kale Ö, Akkar S, Ansari A, Hamzehloo H (2015) A ground-motion predictive model for Iran and Turkey for horizontal PGA, PGV, and 5% damped response spectrum: Investigation of possible regional effects. Bull Seism Soc Am 105:963–980
Kappos A, Pitilakis K, Stylianidis K (1995) Cost-benefit analysis for the seismic rehabilitation of buildings in Thessaloniki, based on a hybrid method of vulnerability assessment. In: Proceedings of the Fifth International Conference on Seismic Zonation, Nice, France, 406–413
Kappos A, Stylianidis K, Pitilakis K (1998) Development of seismic risk scenarios based on a hybrid method of vulnerability assessment. Nat Hazards 17:177–192
Kazantzi A, Vamvatsikos D, Porter K (2015) Analytical seismic vulnerability assessment for a class of modern low-rise steel frames. In: 12th International Conference on Applications of Statistics and Probability in Civil Engineering, ICASP12. Vancouver, Canada
Kennedy R, Ravindra M (1984) Seismic fragilities for nuclear power plant risk studies. Eng Design 79:47–68
Kohrangi M, Bazzurro P, Vamvatsikos D (2016) Vector and scalar IMs in structural response estimation, Part II: Building Demand Assessment. Earthq Spect 32:1525–1543
Kohrangi M, Bazzurro P, Vamvatsikos D, Spillatura A (2017) Conditional spectrum-based ground motion record selection using average spectral acceleration. Earthq Eng Struct Dyn 46:1667–1685
Kohrangi M, Kotha SR, Bazzurro P (2020) Impact of partially non-ergodic site-specific probabilistic seismic hazard on risk assessment of single buildings. Earthq Spect (in-review)
Kohrangi M, Vamvatsikos D, Bazzurro P (2016) Implications of intensity measure selection for seismic loss assessment of 3-D buildings. Earthq Spect 32:2167–2189
Kohrangi M, Vamvatsikos D, Bazzurro P (2017) Site dependence and record selection schemes for building fragility and regional loss assessment. Earthq Eng Struct Dyn 46:1625–1643
Kotha SR, Bindi D, Cotton F (2016) Partially non-ergodic region specific GMPE for Europe and Middle-East. Bull Earthq Eng 14:1245–1263
Kotha SR, Bindi D, Cotton F (2017) From ergodic to region- and site-specific probabilistic seismic hazard assessment: method development and application at European and Middle Eastern sites. Earthq Spect 33:1433–1453
Kotha SR, Weatherill G, Bindi D, Cotton F (2020) A regionally adaptable ground-motion model for shallow crustal earthquakes in Europe. Bull Earthq Eng
Landwehr N, Kuehn NM, Scheffer T, Abrahamson N (2016) A nonergodic ground-motion model for california with spatially varying coefficients. Bull Seism Soc Am 106:2574–2583
Lanzano G, Sgobba S, Luzi L, Puglia R, Pacor F, Felicetta C, D’amico M, Cotton F, Bindi D (2018) The pan-European engineering strong motion (ESM) flatfile: compilation criteria and data statistics. Bull Earthq Eng, 1–22
Lin P-S, Chiou B, Abrahamson N, Walling M, Lee C-T, Cheng C-T (2011) Repeatable source, site, and path effects on the standard deviation for empirical ground-motion prediction models. Bull Seism Soc Am 101:2281–2295
Luco N, Cornell C (2007) Structure-specific scalar intensity measures for near-source and ordinary earthquake ground motions. Earthq Spect 23:357–392
Luen B, Stark P (2011) Are declustered earthquake catalogs Poisson. Statistics, 1–8
Marzocchi W, Taroni M (2014) Some thoughts on declustering in probabilistic seismic-hazard analysis. Bull Seism Soc Am 104:1838–1845
Mcguire RK (2004) Seismic hazard and risk analysis. Earthquake Engineering Research Institute
Mitrani-Reiser J (2007) An ounce of prediction: probabilistic loss estimation for performance-based earthquake engineering. PhD Dissertation, Calofornia Institute of Technology
Noh H, Lallemant D, Kiremidjian A (2015) Development of empirical and analytical fragility functions using kernel smoothing methods. Earthq Eng Struct Dyn 44:1163–1180
Ogata Y (1998) Space-time point-process models for earthquake occurrences. Ann Inst Stat Math 50:379–402
Orsini G (1999) A model for buildings’ vulnerability assessment using the parameterless scale of seismic intensity (PSI). Earthq Spect 15:463–483
Papadopoulos A, Bazzurro P (2021) Exploring probabilistic seismic risk assessment accounting for seismicity clustering and damage accumulation: Part II. Risk Analysis, Earthq Spect 37(1):386–408
Pinho R, Bommer J, Glaister SA (2002) Simplified approach to displacement-based earthquake loss estimation analysis. In: Proceedings of the 12th European Conference on Earthquake Engineering, London, UK: Paper No. 738 (on CD)
Porter K, Farokhnia K, Vamvatsikos D, Cho I (2014) Guidelines for component-based analytical vulnerability assessment of buildings and nonstructural elements. GEM Technical Report 2014–13: Global Earthquake Model Foundation, Pavia, Italy
Porter K, Kennedy R, Bachman R (2007) Creating fragility functions for performance-based earthquake engineering. Earthq Spect 23:471–489
Porter K, Kiremidjian A, Legrue J (2001) Assembly-based vulnerability of buildings and its use in performance evaluation. Earthq Spect 17:290–312
Radiguet M, Cotton F, Manighetti I, Campillo M, Douglas J (2009) Dependency of near-field ground motions on the structural maturity of the ruptured faults. Bulle Seism Soc Am 99:2572–2581
Restrepo-Vélez L, Magenes G (2004) Simplified procedure for the seismic risk assessment of unreinforced masonry buildings. In: Proceedings of the 13th World Conference on Earthquake Engineering, Vancouver, Canada
Rodriguez-Marek A, Cotton F, Abrahamson NA, Akkar S, Al Atik L, Edwards B, Montalva GA, Dawood HM (2013) A model for single-station standard deviation using data from various tectonic regions. Bull Seism Soc Am 103:3149–3163
Rossetto T, Elnashai A (2003) Derivation of vulnerability functions for european-type RC structures based on observational data. Eng Struct 25:1241–1263
Rossetto T, Ioannou I, Grant D, Maqsood T (2014) Guidelines for empirical vulnerability assessment. GEM Technical Report, GEM Foundation, Pavia
Sextos A, Risi R, Pagliaroli A, Foti S, Passeri F, Ausilio E, Cairo R, Capatti MC, Chiabrando F, Chiaradonna A, Dashti S, De Silva F, Dezi F, Durante M, Giallini S, Lanzo G, Sica S, Simonelli AL, Zimmaro P (2018) Local site effects and incremental damage of buildings during the 2016 Central Italy Earthquake sequence. Earthq Spect 34
Shokrabadi M, Burton HV (2017) Building service life economic loss assessment under sequential seismic events. Earthq Eng Struct Dyn 47
Shokrabadi M, Burton HV (2019) Regional short-term and long-term risk and loss assessment under sequential seismic events. Eng Struct 185:366–376
Silva V, Crowley H, Varum H, Pinho R, Sousa L (2015) Investigation of the characteristics of Portuguese regular moment-frame RC buildings and development of a vulnerability model. Bull Earthq Eng 13:1455–1490
Silva V, Crowley H, Varum H, Pinho R, Sousa R (2014) Evaluation of analytical methodologies used to derive vulnerability functions. Earthq Eng Struct Dyn 43:181–204
Sousa M, Costa AC, Carvalho A, Coelho E (2004) An automatic seismic scenario loss methodology integrated on a geographic information system. In: Proceedings of the 13th World Conference on Earthquake Engineering, Vancouver, Canada. Paper No. 2526 (on CD)
Stewart J, Lanzo G, Ausilio E, Cairo R, Bozzoni, F, Capatti MC, Pasqua F, Dezi F, Di Sarno L, Durante M, Simonelli AL, Foti S, Chiabrando F, Dabove P, Di Pietra V, Maschio P, Passeri F, Sgobio A, Teppati Losè L, Zimmaro P (2017a) Engineering reconnaissance following the October 2016 Central Italy Earthquakes
Stewart JP, Afshari K, Goulet CA (2017) Non-ergodic site response in seismic hazard analysis. Earthq Spect 33:1385–1414
Stiphout T, Schorlemmer D, Wiemer S (2011) The effect of uncertainties on estimates of background seismicity rate. Bull Seism Soc Am 101:482–494
Straub D, Der Kiureghian A (2008) Improved seismic fragility modeling from empirical data. Struct Safety 30:320–336
Tothong P, Luco N (2007) Probabilistic seismic demand analysis using advanced ground motion intensity measures. Earthq Eng Struct Dyn 36:1837–1860
Vamvatsikos D, Cornell C (2002) Incremental dynamic analysis. Earthq Eng Struct Dyn 31:491–514
Vamvatsikos D, Cornell C (2005) Developing efficient scalar and vector intensity measures for IDA capacity estimation by incorporating elastic spectral shape information. Earthq Eng Struct Dyn 34:1573–1600
Villar-Vega M, Silva V, Crowley H, Yepes C, Tarque N, Acevedo AB, Hube MA, Gustavo CD, María HS (2017) Development of a fragility model for the residential building stock in South America. Earthq Spect 33:581–604
Walling MA (2009) Non-ergodic probabilistic seismic hazard analysis and spatial simulation of variation in ground motion. University of California, Berkeley
Weatherill G, Kotha SR, Cotton F (2020) A Regionally-adaptable “scaled-backbone” ground motion logic tree for shallow seismicity in Europe: application in the 2020 European seismic hazard model. Bull Earthq Eng
Weatherill G, Pagani M, Garcia J (2016) Exploring earthquake databases for the creation of magnitude-homogeneous catalogues: tools for application on a regional and global scale. Geophysical J Int 206:1652–1676
Woessner J, Laurentiu D, Giardini D, Crowley H, Cotton F, Grünthal G, Valensise G, Arvidsson R, Basili R, Demircioglu MB, Hiemer S, Meletti C, Musson RW, Rovida AN, Sesetyan K, Stucchi M (2015) The 2013 European seismic hazard model: key components and results. Bull Earthq Eng 13:3553–3596
Zhang L, Werner M, Goda K (2018) Spatiotemporal seismic hazard and risk assessment of aftershocks of M9 Megathrust Earthquakes. Bull Seism Soc Am 108:3313–3335
Author information
Authors and Affiliations
Corresponding author
Editor information
Editors and Affiliations
Rights and permissions
Open Access This chapter is licensed under the terms of the Creative Commons Attribution 4.0 International License (http://creativecommons.org/licenses/by/4.0/), which permits use, sharing, adaptation, distribution and reproduction in any medium or format, as long as you give appropriate credit to the original author(s) and the source, provide a link to the Creative Commons license and indicate if changes were made.
The images or other third party material in this chapter are included in the chapter's Creative Commons license, unless indicated otherwise in a credit line to the material. If material is not included in the chapter's Creative Commons license and your intended use is not permitted by statutory regulation or exceeds the permitted use, you will need to obtain permission directly from the copyright holder.
Copyright information
© 2021 The Author(s)
About this chapter
Cite this chapter
Kohrangi, M., Papadopoulos, A., Kotha, S.R., Vamvatsikos, D., Bazzurro, P. (2021). Earthquake Catastrophe Risk Modeling, Application to the Insurance Industry: Unknowns and Possible Sources of Bias in Pricing. In: Akkar, S., Ilki, A., Goksu, C., Erdik, M. (eds) Advances in Assessment and Modeling of Earthquake Loss. Springer Tracts in Civil Engineering . Springer, Cham. https://doi.org/10.1007/978-3-030-68813-4_11
Download citation
DOI: https://doi.org/10.1007/978-3-030-68813-4_11
Published:
Publisher Name: Springer, Cham
Print ISBN: 978-3-030-68812-7
Online ISBN: 978-3-030-68813-4
eBook Packages: EngineeringEngineering (R0)